
Laurie Williams
Laurie Williams is a Distinguished University Professor in the Computer Science Department of the College of Engineering at North Carolina State University (NCSU). Laurie is a co-director of the NCSU Science of Security Lablet sponsored by the National Security Agency, the NCSU Secure Computing Institute, and is the Principal Cybersecurity Technologist of the SecureAmerica Institute. Laurie’s research focuses on software security; agile software development practices and processes, particularly continuous deployment; and software reliability, software testing and analysis. Laurie is an ACM and an IEEE Fellow.
Solving Software Security Challenges with Artificial Intelligence
Description
Software security lies at the intersection of software engineering and cybersecurity – building security into a product. Software security techniques focus on preventing the injection of vulnerabilities and detecting the vulnerabilities that make their way into a product or the deployment pipeline before the product is released. Increasingly, artificial intelligence is being used to power software security techniques to aid organizations in deploying secure products. This talk will present a landscape of research and practice at the intersection of software engineering, cybersecurity, and artificial intelligence to solve cybersecurity challenges. The talk will also present research projects conducted by the speaker’s own research group.

Leandro L. Minku
Leandro L. Minku is a Senior Lecturer at the School of Computer Science, University of Birmingham (UK). Dr. Minku’s main research interests are machine learning in non-stationary environments / data stream mining, online class imbalance learning, ensembles of learning machines and computational intelligence for software engineering. Among others, Leandro Minku is an Associate Editor-in-Chief of the Neurocomputing journal, Associate Editor of IEEE Transactions on Neural Networks and Learning Systems, Coordinating Editor of Empirical Software Engineering journal and Associate Editor of the Journal of Systems and Software.
Prediction of Defect-Inducing Software Changes in Dynamic Environments
Description
Software systems have become ever larger and more complex. This inevitably leads to software defects, which are costly to debug and fix. To help reducing the number of defects, machine learning approaches have been proposed for predicting defect-inducing changes in software source code at commit time. By inspecting such changes at commit time, developers can reduce the chances of inducing defects that are much more costly to debug and fix at later stages. While results in this field are promising, most existing work assumes that the characteristics of the problem remain the same over time. However, the environment where software projects are conducted is dynamic, being affected by changes in the development team, in the management strategy, in the current stage of the software development, among others. Such dynamism means that the defect generating process can suffer variations over time, impairing the ability of machine learning classifiers to perform well. In this talk, I will discuss the latest advancements in dealing with prediction of defect-inducing software changes in dynamic environments.

Carolyn Seaman
Carolyn Seaman is a Professor of Information Systems at the University of Maryland Baltimore County (UMBC). She is also the Director of the Center for Women in Technology, also at UMBC. Her research consists mainly of empirical studies of software engineering, with particular emphases on maintenance, organizational structure, communication, measurement, and technical debt. She also investigates qualitative research methods in software engineering, as well as computing pedagogy. She holds a PhD in Computer Science from the University of Maryland, College Park, a MS from Georgia Tech, and a BA from the College of Wooster (Ohio).
Decision Making in Software Engineering: The Central Role of Technical Debt
Description
Technical Debt is a metaphor that captures the common tradeoff in software development projects between short-term pressures (e.g. delivery time) and long-term concerns (e.g. maintainability). It refers to the existing liabilities in a software product that were created in response to a short-term pressure but that pose a risk to the ability of the team to maintain the product over time. Common types of technical debt include overly complex code, poorly structured code, undocumented code, degradation of the software architecture, insufficient testing, etc. Decisions about whether to incur debt, or when to pay off debt, or which debt to pay off, perfectly encapsulate the short vs. long term tradeoffs that are typical in software engineering. In fact, it can be argued that nearly all decisions made during a software project are in some way related to Technical Debt. Thus, deeply understanding and improving Technical Debt decision making will have a significant impact on the management of software projects in general. In this keynote, we will review current streams in decision making research, in Technical Debt, in software engineering in general, and in related disciplines.
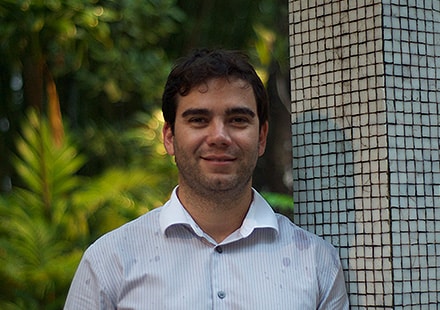
Alessandro Garcia
Dr. Garcia holds a PhD in Computer Science from the Pontifical Catholic University of Rio de Janeiro (2004). He was an Assistant Professor at Lancaster University (England) from February 2005 to January 2009 and served as a member of the Editorial Board of two major international journals in his area: IEEE Transactions on Software Engineering and Journal of Systems and Software. Professor Garcia has been frequently invited to the Program Committees and/or Organization Committees of the major international Software Engineering conferences and related areas such as ICSE, FSE, ICSME, MODELS, ICPC, ESEM, and several others.
From Intuition to Spirituality: What Are their Roles in Software Design?
Description
Intuition is the ability to understand something “automatically”, without the need for conscious reasoning. The use of intuition along software design may have a major impact on its overall quality. Software design is an activity that requires decisions being made all the time by architects, developers, testers, maintainers, reviewers and others. These stakeholders’ intuitions are likely to drive various design decisions given the need for rapidity and many other software project factors. Despite intuition being widely studied in many disciplines, its role in software design has rarely been investigated in depth. One may suspect that intuition, given the sudden design decisions triggered from it, may do more harm than good in software design. However, this is not necessarily the case. We still have limited knowledge about the influence of intuition in software quality. There are different types of intuition being investigated in other disciplines, including more controversial ones, such as “spiritual intuition”. The purpose of this talk is to make a case on the importance of studying the role of certain intuition types in software design. We will present some possible research directions that are likely to enable us to understand whether and how our methods, tools and IDEs could stimulate “positive” intuition in software design.